class: center, middle, inverse, title-slide # Deep Learning e R ## SEST - 2018 ### Daniel Falbel (
@Curso-R
)
dfalbel@curso-r.com
### 24/05/2018 --- ## Oi! - Bacharel em Estatística (2015) - Sócio da [Curso-R](http://curso-r.com) - Sócio da [R6](http://rseis.com.br) --- ## Deep Learning * Redes neurais com muitas camadas. * A partir de 2012 obteve pelas primeiras vezes resultados super-humanos em tarefas de reconhecimento de imagens. * Recentemente alcançou bastante sucesso em problemas de classificação de imagens, audio e texto. 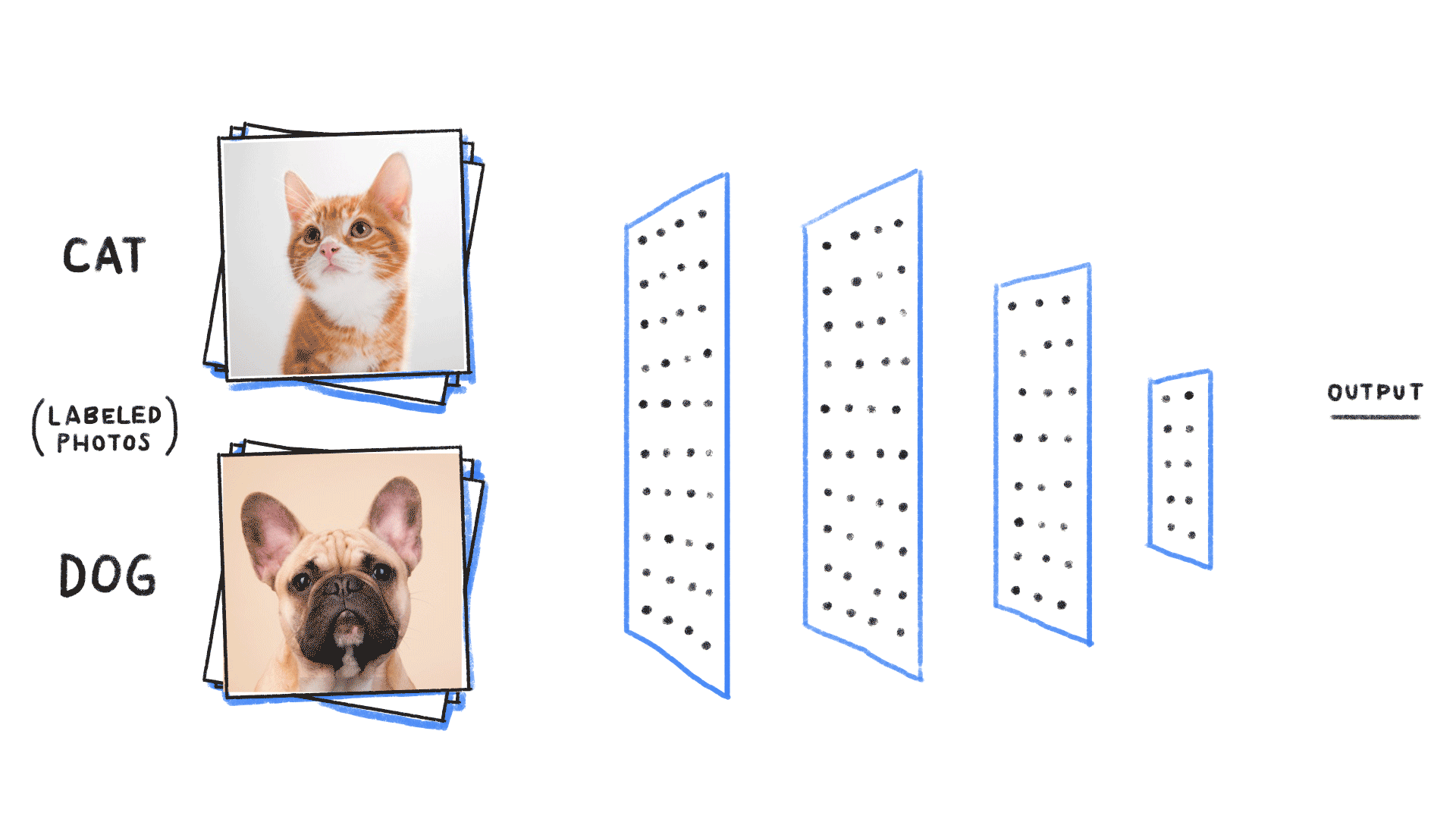 --- ## Algumas aplicações ### [Show and Tell](https://ai.googleblog.com/2016/09/show-and-tell-image-captioning-open.html) 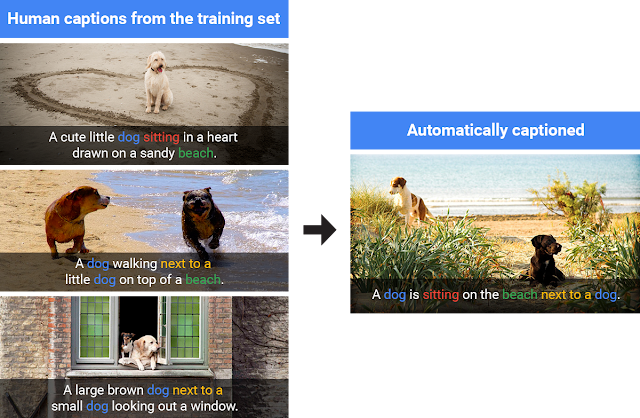 --- ### [Zero Shot Translation](https://ai.googleblog.com/2016/11/zero-shot-translation-with-googles.html) 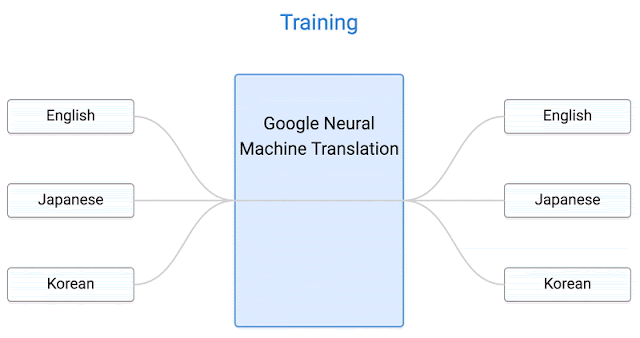 --- ### [Speech Generation](https://google.github.io/tacotron/publications/tacotron2/index.html) 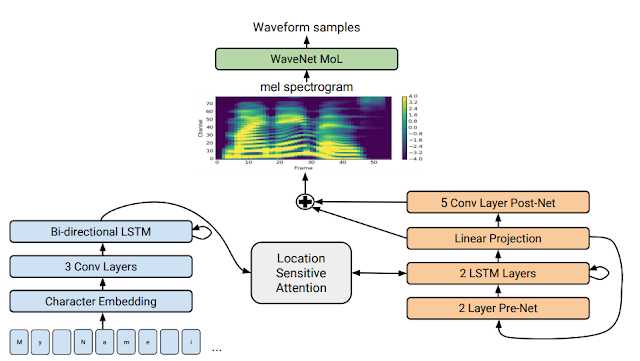 --- ### [The Case for Learned Index Structures](https://arxiv.org/abs/1712.01208) 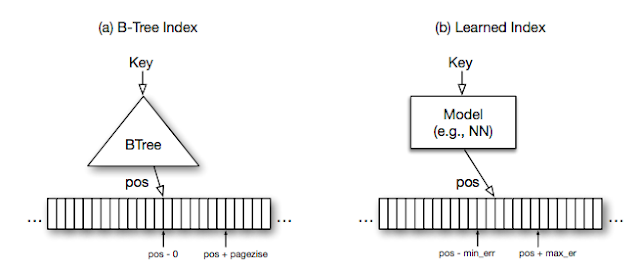 --- class: inverse, middle, center ## Exemplo ### [Classifying duplicate questions from Quora with Keras](https://tensorflow.rstudio.com/blog/keras-duplicate-questions-quora.html) --- ## Keras * Uma API que permite especificar modelos de *Deep Learning* de forma intuitiva e rápida. * Criada por François Chollet (@fchollet).  * Originalmente implementada em `python`. --- ## Keras * Uma API com múltiplas implementações. <!-- --> --- ## Keras + R * Pacote do R: [`keras`](https://github.com/rstudio/keras). * Baseado em [reticulate](https://github.com/rstudio/reticulate). * Desenvolvido pelo JJ Allaire (CEO do RStudio). * Tem uma sintaxe R-like com uso de `%>%`. 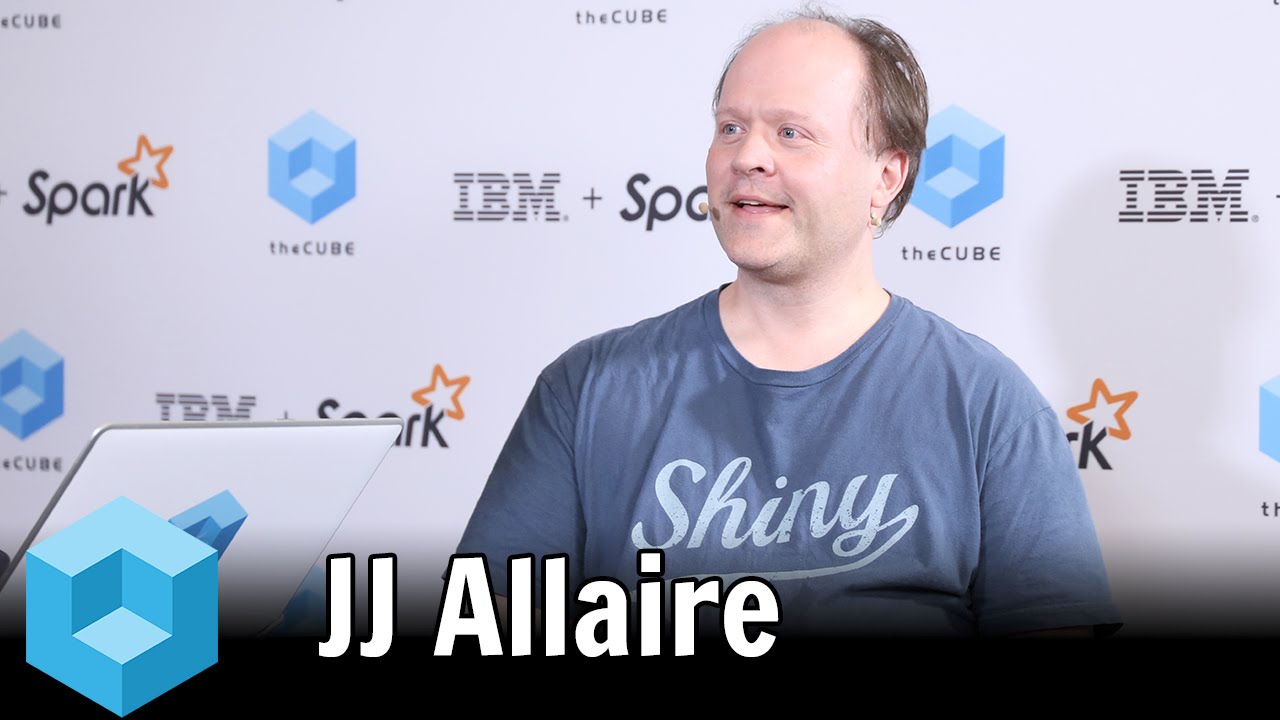 --- 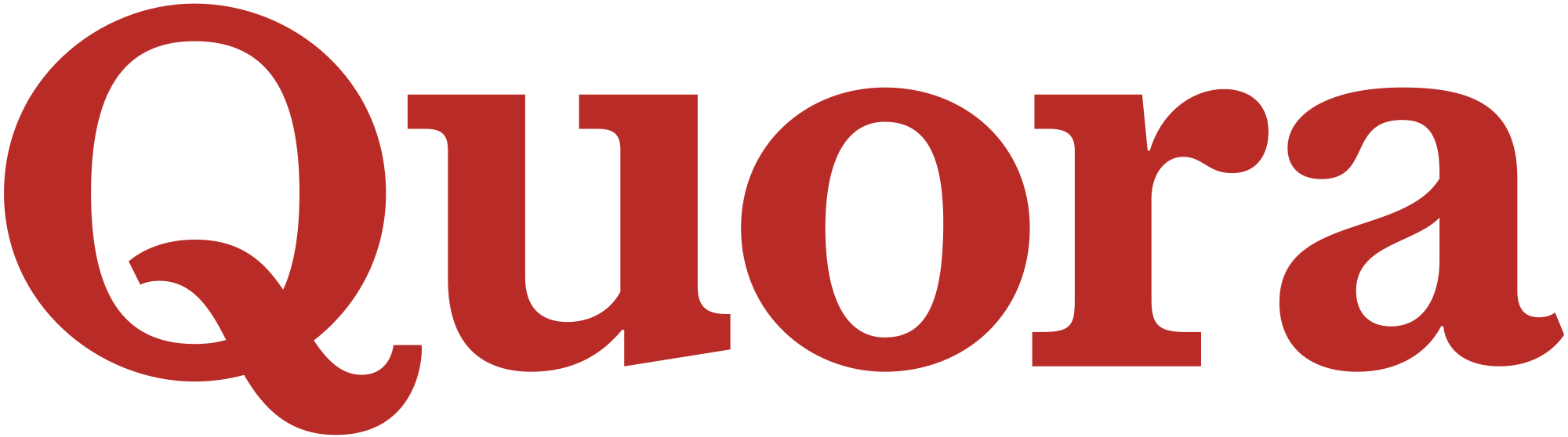 * [Quora](https://www.quora.com): site de perguntas e respostas de âmbito geral. * Para quem usa o Quora, é melhor ter apenas uma versão de uma pergunta. * Banco de dados de uma [competição do Kaggle](https://www.kaggle.com/c/quora-question-pairs). * ~400k pares de perguntas duplicadas (ou não) marcadas pelos moderadores do site. * **Objetivo:** Identificar os pares de perguntas que possuem o mesmo _significado_. * Antes da competição o problema era resolvido com Random Forests, depois passaram a usar [Deep Learning](https://engineering.quora.com/Semantic-Question-Matching-with-Deep-Learning). --- ### Duplicadas <div> .pull-left[ * How can I be a good geologist? * How do I read and find my YouTube comments? * What can make Physics easy to learn? ] .pull-right[ - What should I do to be a great geologist? - How can I see all my Youtube comments? - How can you make physics easy to learn? ] </div> ### Não-Duplicadas <div> .pull-left[ * How can I increase the speed of my internet connection while using a VPN? * What is the step by step guide to invest in share market in india? * How do I get over my ex's past? ] .pull-right[ * How can Internet speed be increased by hacking through DNS? * What is the step by step guide to invest in share market? * What is the best way to get over your ex? ] </div> --- ## Arquitetura do modelo <br> <br> <!-- --> * Siamese LSTM --- #### Embedding <!-- --> --- ## LSTM <!-- --> --- ## Arquitetura no Keras <br> <br> <!-- --> --- ## Código ```r library(keras) ``` -- ```r input1 <- layer_input(shape = c(20), name = "input_question1") input2 <- layer_input(shape = c(20), name = "input_question2") ``` -- ```r word_embedder <- layer_embedding( input_dim = 50000, # vocab size output_dim = 128, # hyperparameter - embedding size input_length = 20 # padding size ) ``` -- ```r seq_embedder <- layer_lstm(units = 128) ``` -- ```r vector1 <- input1 %>% word_embedder() %>% seq_embedder() vector2 <- input2 %>% word_embedder() %>% seq_embedder() ``` -- ```r #> Tensor("lstm_1/TensorArrayReadV3:0", shape=(?, 128), dtype=float32) #> Tensor("lstm_1_1/TensorArrayReadV3:0", shape=(?, 128), dtype=float32) ``` --- ## Arquitetura do Modelo <br> <br> <!-- --> --- ## Código ```r cosine_similarity <- layer_dot(list(vector1, vector2), axes = 1) ``` -- ```r output <- cosine_similarity %>% layer_dense(units = 1, activation = "sigmoid") ``` -- ```r model <- keras_model(list(input1, input2), output) model %>% compile( optimizer = "adam", metrics = list(acc = metric_binary_accuracy), loss = "binary_crossentropy" ) ``` --- ```r summary(model) ``` ```r # _______________________________________________________________________________________ # Layer (type) Output Shape Param # Connected to # ======================================================================================= # input_question1 (InputLayer (None, 20) 0 # _______________________________________________________________________________________ # input_question2 (InputLayer (None, 20) 0 # _______________________________________________________________________________________ # embedding_1 (Embedding) (None, 20, 128) 6400256 input_question1[0][0] # input_question2[0][0] # _______________________________________________________________________________________ # lstm_1 (LSTM) (None, 128) 131584 embedding_1[0][0] # embedding_1[1][0] # _______________________________________________________________________________________ # dot_1 (Dot) (None, 1) 0 lstm_1[0][0] # lstm_1[1][0] # _______________________________________________________________________________________ # dense_1 (Dense) (None, 1) 2 dot_1[0][0] # ======================================================================================= # Total params: 6,531,842 # Trainable params: 6,531,842 # Non-trainable params: 0 # _______________________________________________________________________________________ ``` --- ## Treinando ```r model %>% fit( list(train_question1_padded, train_question2_padded), train_is_duplicate, batch_size = 64, epochs = 10, validation_data = list( list(val_question1_padded, val_question2_padded), val_is_duplicate ) ) ``` ```r # Train on 363861 samples, validate on 40429 samples # Epoch 1/10 # 363861/363861 [==============================] - 89s 245us/step - loss: 0.5860 - acc: 0.7248 - val_loss: 0.5590 - val_acc: 0.7449 # Epoch 2/10 # 363861/363861 [==============================] - 88s 243us/step - loss: 0.5528 - acc: 0.7461 - val_loss: 0.5472 - val_acc: 0.7510 # Epoch 3/10 # 363861/363861 [==============================] - 88s 242us/step - loss: 0.5428 - acc: 0.7536 - val_loss: 0.5439 - val_acc: 0.7515 ``` --- class: inverse, middle, center ## Predições com Shiny [Shiny](https://jjallaire.shinyapps.io/shiny-quora/) --- ## Mais * [Galeria de exemplos do Keras](https://keras.rstudio.com/articles/examples/index.html). * [Livro: Deep Learning com R](https://www.amazon.com/Deep-Learning-R-Francois-Chollet/dp/161729554X) * [Blog: Tensorflow for R](https://tensorflow.rstudio.com/blog.html) 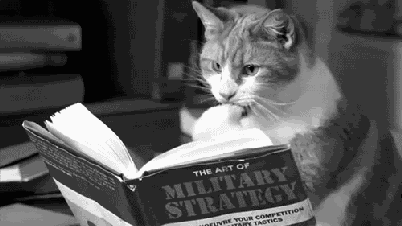 --- class: inverse, middle, center ## Obrigado dfalbel@curso-r.com github.com/dfalbel sest.curso-r.com